It is important to notice that, while the McClelland and Rummelhart model performed a
mapping from word forms to word forms, the model by Gasser and Lee performed a mapping
from words forms to meanings, and from meanings to word forms. Their network used between
16 and 20 hidden nodes, and managed to cirrectly produce the past tense of input verbs in 95.5%
of all training data. The networks, though, were not good at generalizing for new data. |
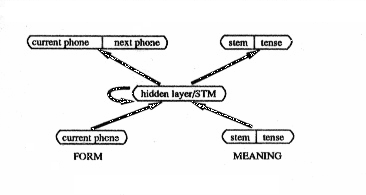 Figure 7: Sketch of the network used by
Gasse & Lee. From A Short-Term Memory Architecture for the Learning of Morphophonemic Rules. |